Revolutionizing Image Understanding: The Power of Automatic Image Annotation
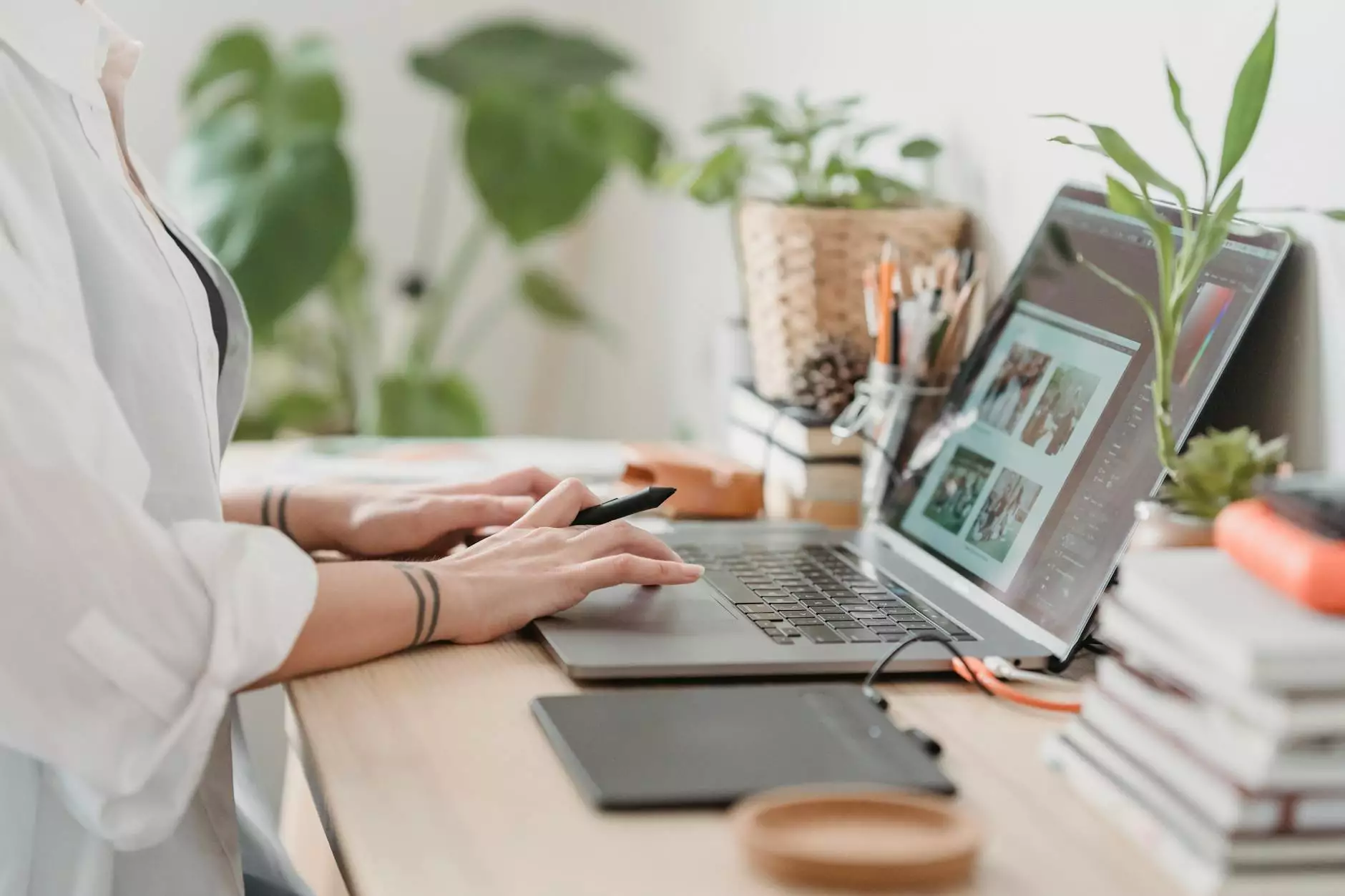
In the rapidly evolving world of technology, the need for precise and efficient data processing has never been more crucial. One area that has seen significant advancements is automatic image annotation, which plays a pivotal role in enabling machines to understand and categorize visual data accurately. This article delves into the intricacies of this technology, its applications, and how it can benefit businesses today.
Understanding Automatic Image Annotation
Automatic image annotation refers to the process by which a machine learning model labels an image with corresponding tags that describe its content. This technology leverages advanced algorithms, primarily through deep learning, to identify objects, scenes, and attributes within images. The primary goal is to reduce the manual effort involved in image labeling while improving accuracy and consistency.
The Importance of Automatic Image Annotation in Modern Business
In the context of business, the ability to analyze and categorize vast amounts of visual data is invaluable. Here are a few reasons why automatic image annotation is becoming increasingly essential:
- Efficiency: Manual annotation can be time-consuming and labor-intensive. Automating this process allows businesses to focus on core activities rather than spending resources on repetitive tasks.
- Scalability: As the volume of visual data increases, businesses require a solution that can scale with their needs. Automatic image annotation can handle vast datasets, allowing for greater analytical depth.
- Accuracy: Algorithms trained on large datasets can often outperform human annotators in terms of consistency and accuracy, drastically reducing the chances of error.
- Cost Savings: By minimizing the time and manpower required for image annotation, businesses can significantly reduce operational costs.
Applications of Automatic Image Annotation
The applications of automatic image annotation are vast and varied. Here are some key industries reaping the benefits:
1. E-commerce
In the e-commerce sector, businesses leverage automatic image annotation to enhance product categorization. By tagging images with relevant details, companies can improve their search functions, enabling customers to find products quickly and efficiently.
2. Healthcare
The healthcare industry employs automatic image annotation for medical imaging. By annotating scans and images (such as X-rays, MRIs, and CT scans), medical practitioners can more easily identify anomalies, aiding in diagnosis and treatment planning.
3. Autonomous Vehicles
In the realm of self-driving cars, automatic image annotation is crucial for object detection and scene understanding. Annotated images provide the training data needed to help vehicles recognize pedestrians, other vehicles, and road signs, ensuring safer navigation.
4. Social Media Platforms
Social media companies use automatic image annotation to enhance user-generated content tagging. By automating the tagging of images uploaded by users, platforms can curate content more effectively and improve user experience.
5. Security and Surveillance
Automatic image annotation is also vital in security applications. Surveillance systems can use this technology to automatically label and categorize security footage, helping security personnel to identify potential threats quickly.
Key Technologies Behind Automatic Image Annotation
The backbone of automatic image annotation lies in several advanced technologies:
1. Deep Learning
Deep learning algorithms, particularly Convolutional Neural Networks (CNNs), are extensively used in image recognition tasks. These models can analyze image features hierarchically, enabling precise classification and annotation.
2. Natural Language Processing (NLP)
To generate meaningful labels, automatic image annotation systems often integrate NLP techniques. This includes understanding and generating descriptions for images, enhancing the contextual relevance of the annotations.
3. Transfer Learning
Transfer learning allows models trained on large datasets (like ImageNet) to be fine-tuned for specific applications without the need for vast amounts of labeled data, making it easier for businesses to implement automatic annotation solutions.
Challenges in Automatic Image Annotation
While the benefits are substantial, there are challenges in the adoption of automatic image annotation:
- Data Quality: The effectiveness of automatic image annotation relies heavily on the quality of training data. Poorly labeled datasets can lead to inaccurate annotations.
- Contextual Understanding: Most algorithms struggle with contextual nuances in images, which can lead to misinterpretation of the content.
- Computational Resources: Training deep learning models requires significant computational power and resources, which may be a barrier for smaller businesses.
Choosing the Right Data Annotation Tool or Platform
When considering a move towards automatic image annotation, choosing the right tool or platform, like those offered by keylabs.ai, is crucial. Here are some factors to consider:
- Features: Look for platforms that offer a comprehensive set of features, including support for various annotation types and integration capabilities with existing workflows.
- User-Friendliness: A user-friendly interface will enhance adoption rates among team members, facilitating smoother operations.
- Scalability: Ensure the platform can grow with your business, handling larger datasets as your needs evolve.
- Support and Community: Opt for platforms with strong support mechanisms and an engaged community for troubleshooting and best practices.
Future Trends in Automatic Image Annotation
The future of automatic image annotation looks promising. Trends to watch include:
- Integration of AI and ML: The ongoing evolution of artificial intelligence and machine learning will lead to even more sophisticated annotation technologies.
- Increased Demand for Real-Time Processing: As businesses move towards real-time analytics, the need for immediate image annotation will grow, pushing the development of faster algorithms.
- Cross-Platform Compatibility: The future will likely see more tools that integrate seamlessly across different platforms, enhancing workflow efficiency.
Conclusion
In conclusion, automatic image annotation is transforming the landscape of data processing across various industries. By leveraging this technology, businesses can achieve greater efficiency, accuracy, and scalability in image analysis. As advancements continue to drive the development of annotation tools and platforms, companies like keylabs.ai are well-positioned to lead the charge in harnessing the full potential of this technology. As industries increasingly recognize the value of automated processes, the future of image annotation seems bright, promising to unlock new possibilities in data-driven decision-making.
By embracing automatic image annotation, companies not only enhance their operational efficiency but also pave the way for innovation and growth in an increasingly visual world.